The first half of 2024 has witnessed an unprecedented acceleration in AI development and adoption. In our view, major model releases such as OpenAI’s GPT-4o and Google’s Gemini 1.5 have pushed the boundaries of AI capabilities, enabling more sophisticated multimodal interactions and enhanced performance. On the open-source front, as we see it, Meta's Llama 3 pushed the boundaries of what freely available models can achieve.
These advancements have been matched by notable financial milestones: OpenAI reportedly reached a $2 billion annual revenue run rate, while Anthropic and Mistral have raised significant funding rounds at roughly $18 billion and $6 billion valuations, respectively. Meanwhile, AI integration in products has become ubiquitous, with Microsoft's AI-enhanced Office suite and Google's AI-powered search leading the charge – and a hotly-anticipated integration of OpenAI models into Apple iOS to come later this year.
As we reflect on the first half of 2024, it's clear that artificial intelligence has moved from a buzzword to a fundamental force reshaping the startup landscape. The past six months have witnessed not only groundbreaking technological advancements but also a significant shift in how startups approach and implement AI solutions. To understand this impact, we surveyed over seventy portfolio companies across various sectors and stages to provide a detailed snapshot of this rapidly changing landscape.
The State of AI Adoption: Enthusiasm Meets Reality
The startup world has embraced AI with remarkable enthusiasm. An impressive 90% of portfolio companies surveyed are currently investing in AI initiatives, with the healthcare sector leading the charge at a 100% adoption rate. However, this interest hasn't yet fully translated into production deployments. Only 54% of companies have AI solutions in production, highlighting the gap between experimentation and real-world implementation.
We observed this gap between investment and deployment is particularly pronounced in the healthcare sector. Despite their high adoption rate, we noticed healthcare companies are the slowest to deploy AI solutions into production, with a long tail of respondents planning to go into production with their solutions as far out as the second half of 2025. This cautious approach we believe likely stems from the stringent regulatory environment and critical nature of healthcare applications. It’s a stark reminder that in some industries, the stakes are too high for a “move fast and break things” approach.
Interestingly, the survey reveals a direct correlation between company size and AI deployment rate. The survey found that larger companies are more likely to have AI solutions already in production – particularly those with over $100 million in revenue, with 73% already in production. We think this suggests that resources and established infrastructure play a crucial role in moving from experimentation to implementation. Larger companies are more likely to have unallocated slush (or “innovation”) funds, or a greater ability to reallocate existing resources towards AI initiatives.
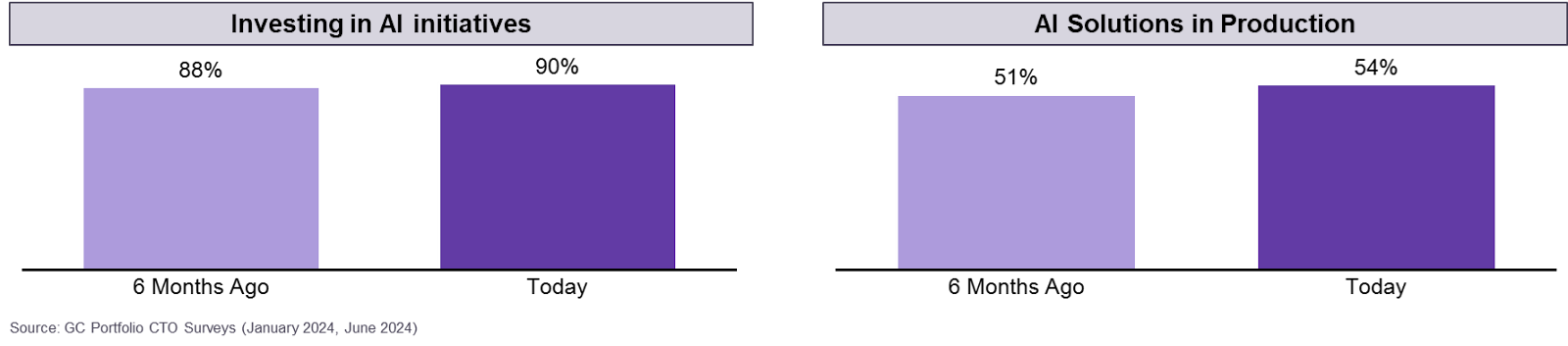
The AI Toolkit: A Diversifying Landscape
When it comes to choosing AI tools, OpenAI continues to dominate the field, with its models used by 89% of portfolio companies surveyed (compared to 76% six months ago). However, the AI ecosystem is far from a monopoly. Anthropic's models, including the newly released Claude 3 family, are gaining traction, used by 17% of respondents. Open-source options like Meta's Llama 2 are also making inroads, with 15% of startups leveraging these models.
This diversification trend is particularly pronounced among pre-revenue companies, who are the most likely to be building models from scratch (30%) and using Anthropic’s models (40%). In our view, this suggests that early-stage startups are more willing to experiment with different approaches to AI, possibly due to fewer legacy constraints.
Interestingly, enterprise-focused companies are the least likely to use OpenAI models (81% compared to the 89% average), indicating to us that different sectors may have different AI needs and preferences.

Shifting Focus: Internal Processes Take Center Stage
Perhaps the most significant shift as we see it, over the past six months has been in how startups are applying AI. While much of the public discourse focuses on customer-facing AI applications, our portfolio companies are increasingly turning their attention inward. The survey reveals a clear prioritization of AI for streamlining internal processes over customer-facing solutions. Specifically:
- 39% of respondents cite internal process optimization as a top focus area for AI
- The second-most cited priority was customer-facing chatbots and virtual assistants, with 30% prioritizing this use case
- A mere 22% are focused on using AI to enable software coding in product development
- Only 20% are prioritizing AI for internal decision support and business intelligence
This pivot towards internal applications makes sense from a practical standpoint. By focusing on internal efficiencies, we believe startups can improve their operations, reduce costs, and potentially deliver better products and services to customers indirectly. Additionally, internal applications may be low-hanging fruit to free up budget to later invest in a wider set of use cases. We think it's a pragmatic approach that promises more immediate and measurable returns on AI investments.

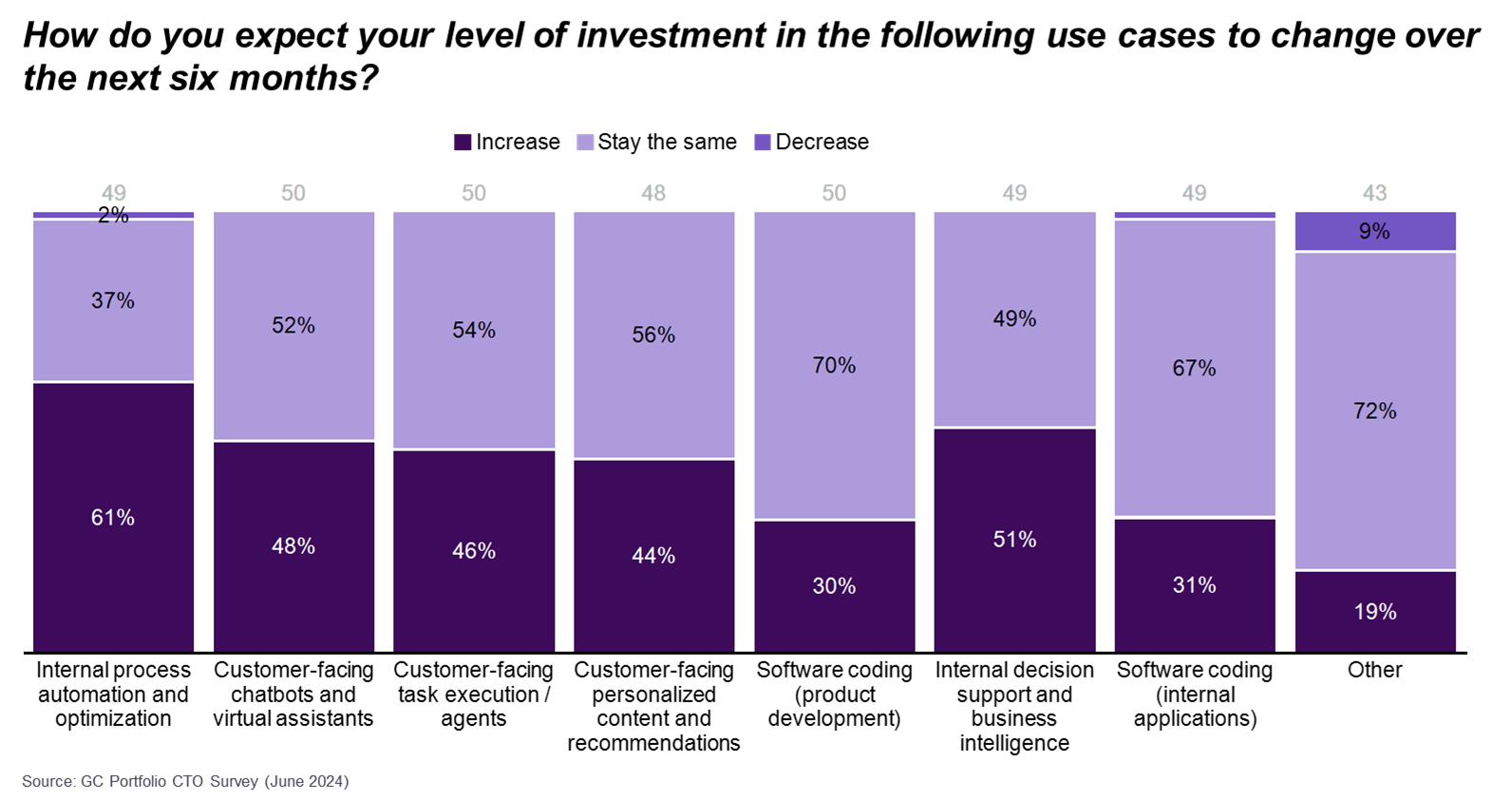
The Investment Surge
The commitment to AI isn't just talk – our portfolio companies are putting their money where their mouth is. As we saw from the survey, on average, companies expect to increase their AI investments by around 1.5x in the next six months compared to the prior six months. We believe this acceleration in spending suggests that many startups are moving from the experimentation phase to more serious, scaled implementations of AI. In particular:
- 61% of respondents plan to increase spending on internal process optimization in the next six months, up from 50% in the previous period
- 51% expect to increase investment in internal decision support and business intelligence applications – a use case which is among the lowest-polling priorities today, with only 20% focused on it today
- Large companies ($100M+ revenue) are expanding AI spend the fastest and across the widest range of use cases, with over 40% of respondents expecting to expand spending on solutions addressing: internal process optimization, internal decision support and BI, customer-facing chatbots, and customer-facing content and recommendations
Employee adoption of AI tools is also set to surge, with an expected 40% growth in the second half of 2024. Currently, an average of 42% of employees are using LLM-based AI tools across the surveyed companies – up from 27% six months ago. This is projected to reach 59% by the end of 2024, indicating that AI is becoming more deeply integrated into day-to-day operations across all levels of organizations.
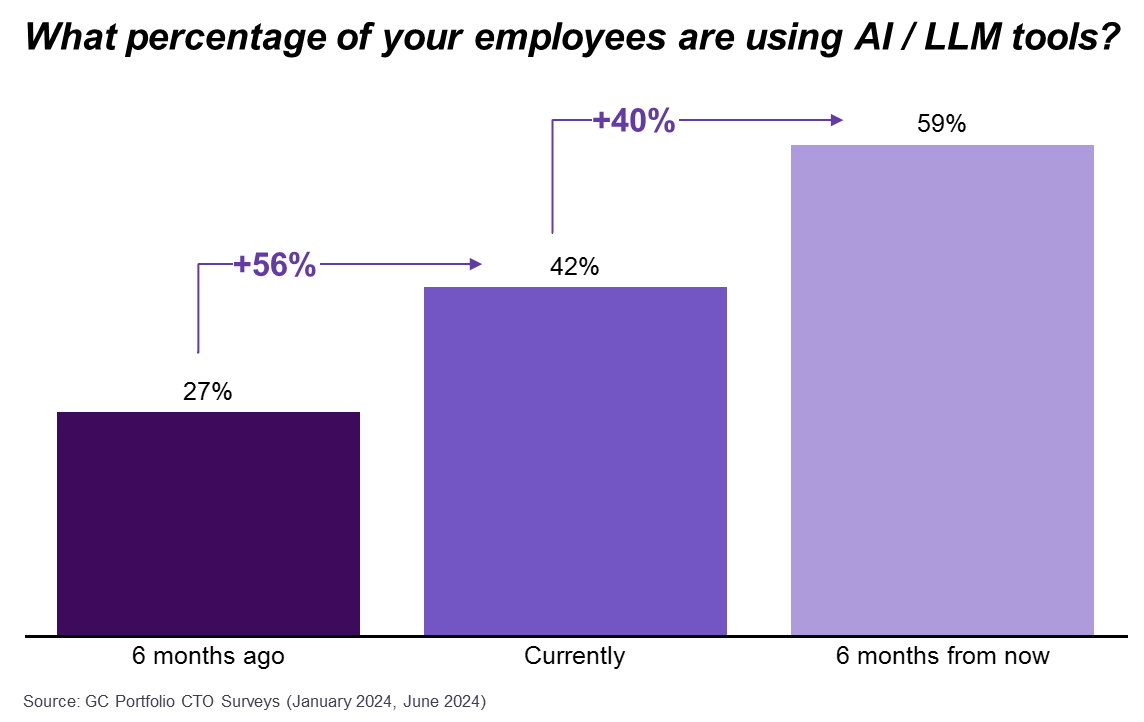
Measuring Success: The ROI Conundrum
As AI investments ramp up, a crucial question emerges: How do we measure the return on these investments? The survey reveals a lack of consensus on this front, with various metrics considered important by a majority of respondents:
- 63% of respondents rate operational efficiency gains as “very important”
- 59% place high importance on customer satisfaction metrics
- 54% prioritize cost reduction as a key indicator
- 52% prioritize revenue growth as a very important metric
Interestingly, different sectors prioritize different metrics. We found Enterprise-focused companies place equal importance on customer satisfaction and revenue growth, while healthcare companies are most focused on tracking productivity gains. This diversity in KPIs reflects the multifaceted impact of AI on businesses. It also highlights the need for startups to develop clear, context-specific metrics for evaluating their AI initiatives.
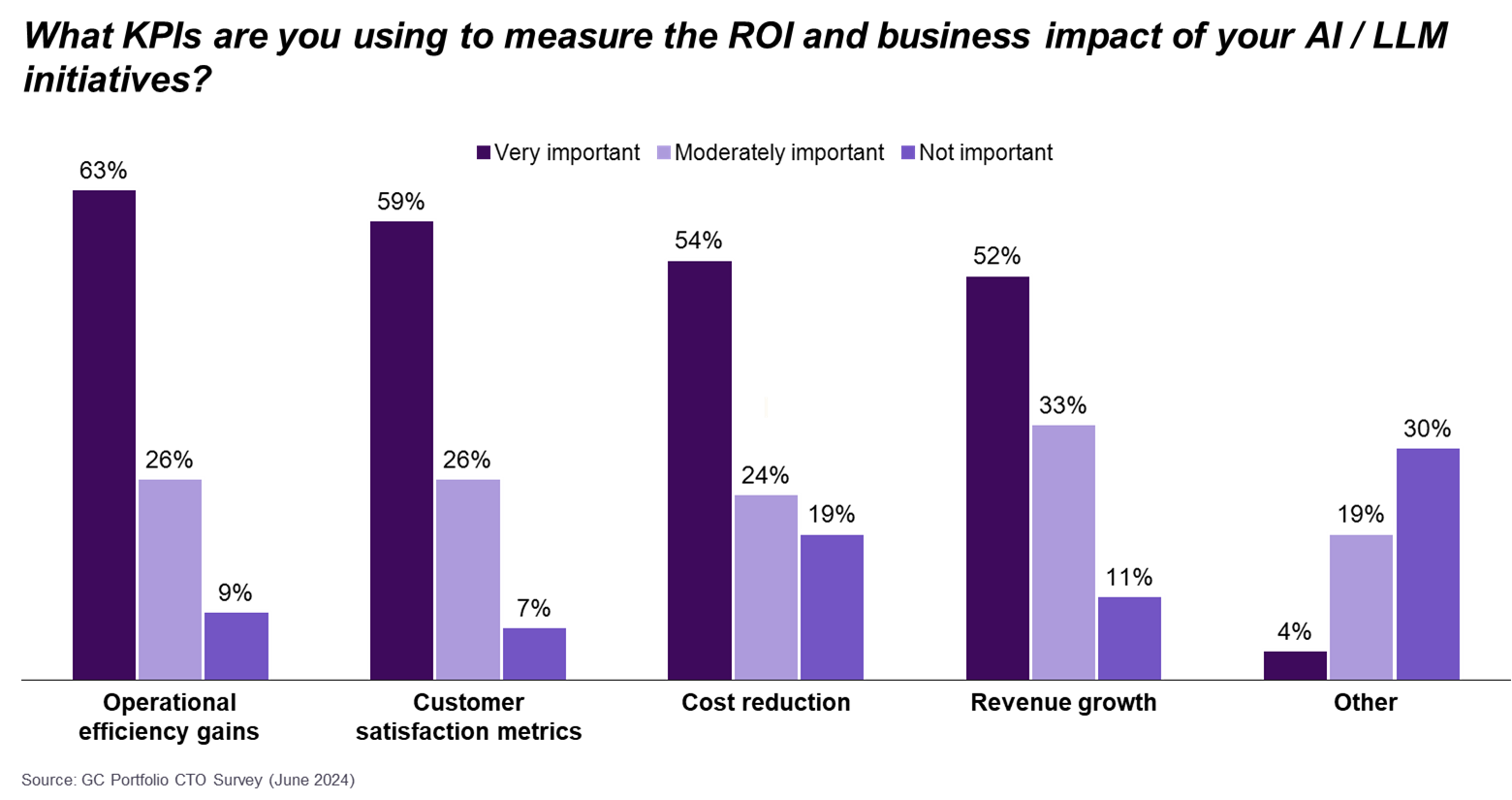
Challenges and Growing Pains: The Road Ahead
Despite the enthusiasm and rate of investment, the path to operationalizing these AI investments isn't without its hurdles. The survey highlights several key challenges:
- Garbage In, Garbage Out: 52% of respondents struggle with data quality and availability issues, underscoring the critical role of data quality for integrating LLMs into organizations – as previously discussed by our colleagues in the piece How AI Could Help Solve the Enterprise Data Quality Dilemma
- Technical Debt: An equal number (52%) cited integration with existing systems as the biggest obstacle to implementing and scaling AI solutions. Surprisingly, this issue is not particularly pronounced in larger or more established companies (56% of pre-revenue compared to 50% of $100M+ revenue respondents)
- Regulatory Concerns: Surprisingly, while 46% cite regulatory issues as their primary challenge, companies in heavily regulated sectors like healthcare are in-line with the cross-sector average. Healthcare companies cite regulatory concerns as secondary to challenges integrating with existing systems – an issue which is not entirely unrelated
- Talent Shortages: 28% of companies point to a lack of skilled personnel as a major barrier. This is particularly pronounced with pre-revenue companies (56%)
These challenges underscore the need for thoughtful, strategic approaches to AI implementation. We believe It's not enough to simply invest in AI; companies need to prepare their infrastructure, define clear success metrics, and ensure they have the right talent to drive their AI initiatives forward.